Results for
I want to build a neural network that takes a matrix A as input and outputs a matrix B such that a constant C=f(A,B)is maximized as much as possible.(The function f()is a custom complex computation function involving random values,probability density,matrix norms,and a series of other calculations).
I tried to directly use 1/f(A,B)or-f(A,B)as the loss function,but I encountered an error stating:"The value to be differentiated is not tracked.It must be a tracked real number dlarray scalar.Use dlgradient to track variables in the function called by dlfeval."I suspect this is likely because f(A,B)is not differentiable.
However,I've also seen people say that no matter what function it is,the dlgradient function can differentiate it.
So,I'm not sure whether it's because the function f()is too complex to be used as a loss function to calculate gradients,or if there's an issue with my code.
If I can't directly use its reciprocal or negative as the loss function,how should I go about training this neural network?Currently,I only know how to implement:providing target values and using functions like mse or huber as loss functions.

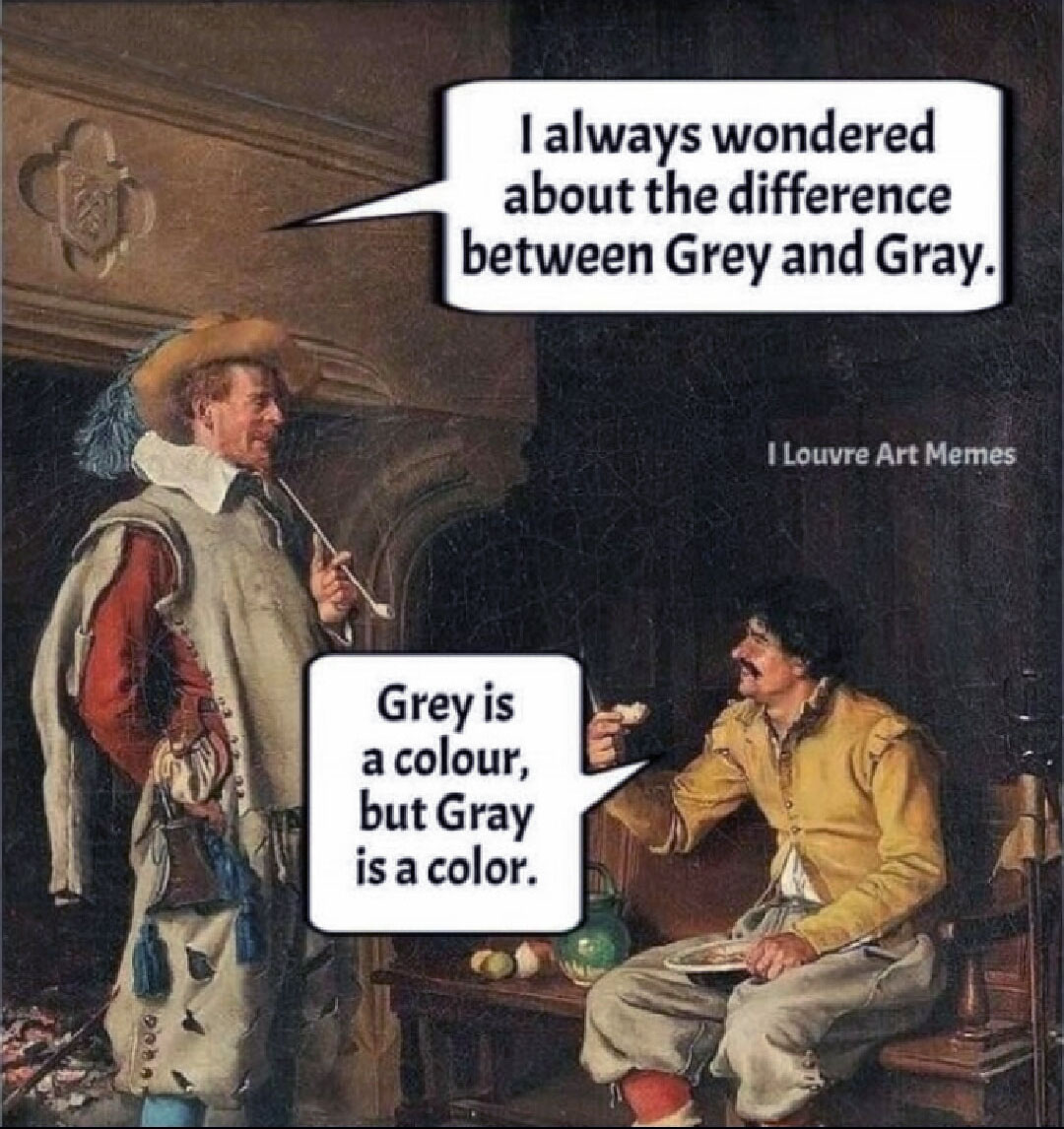
- Channel sounding (for accurate distance measurements)
- Decision-based advertising filtering (for more efficient channel scanning)
- Monitoring advertisers (for improved energy efficiency when devices come into and go out of range)
- Frame space updates (for both higher throughput and better coexistence management)
- Keyless vehicle entry, performed by communication between a key fob or phone and the car’s anchor points
- Smart locks, to permit access only when an authorized device is within a designated proximity to the locks
- Geofencing, to limit access to designated areas
- Warehouse management, to monitor inventory and manage logistics
- Asset tracking for virtually any object of interest

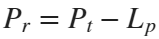
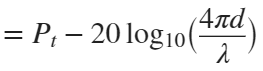
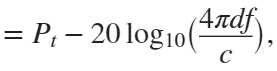








- Round-trip time (RTT) measurement
- Phase-based ranging (PBR) measurement

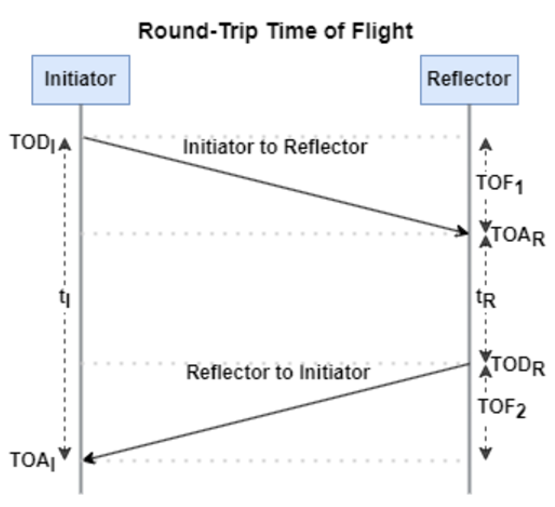









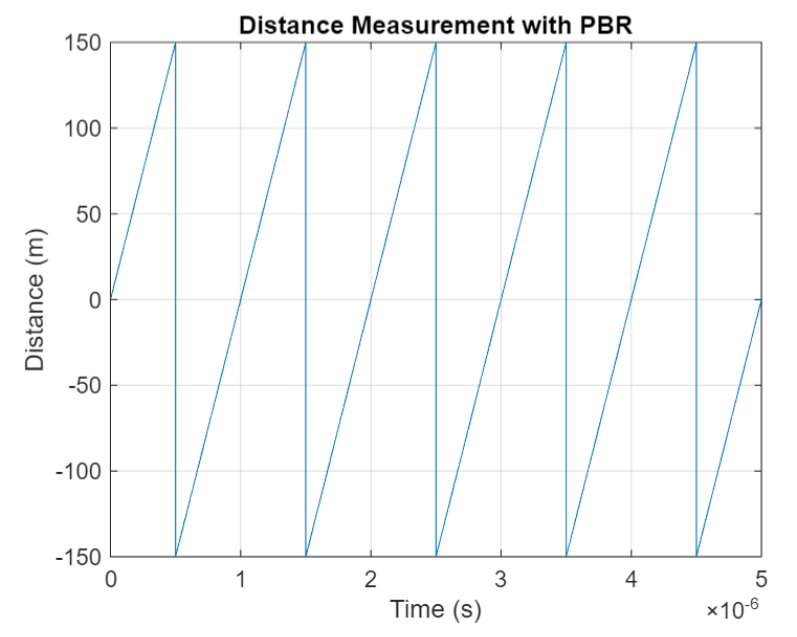
- High Data Throughput, up to 8 Mbps
- 5 and 6 GHz operation
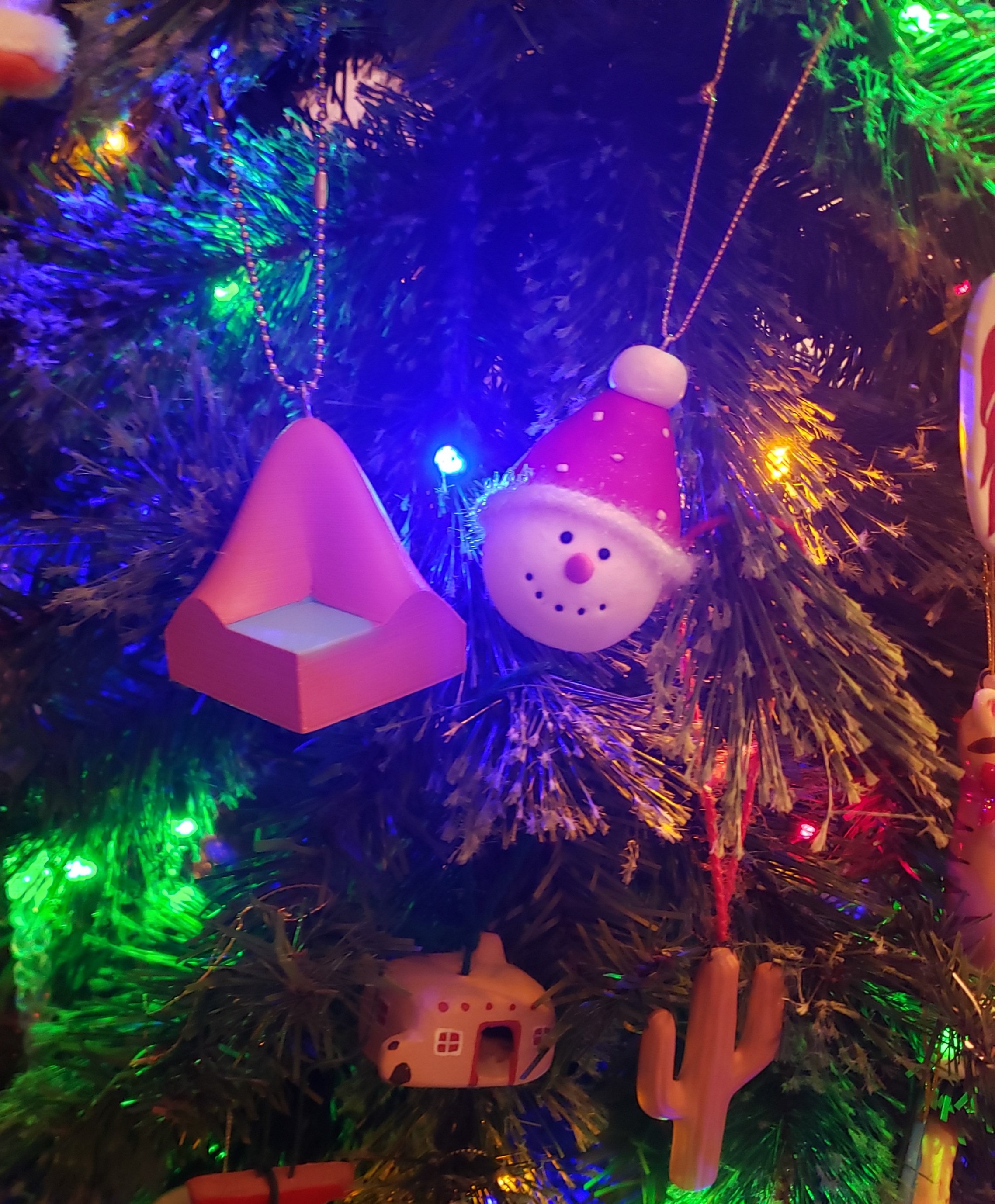
- Parallel Implementation:On my local desktop, I have Number of workers: 6, which means I can evaluate 6 members of the population simultaneously. However, I want to make my code generic so that it automatically adjusts to the number of workers available on any machine. How can I achieve this in MATLAB?
- Improving Convergence Speed:Another approach I’ve come across is using the MigrationInterval and MigrationFractionoptions to divide the population into smaller "islands" that exchange solutions periodically. Would this approach be suitable in my case, and how can I implement it effectively?
- Objective Function Parallelization:Below is the current version of my objective function, which works without parallelization. It writes input variables to an ANSYS .inp file, runs the simulation, and reads results from a text file. How should I modify this function (if needed) to make it compatible with MATLAB’s parallel computing features?
- How to enable and configure parallel computing for GA in MATLAB.
- Any adjustments required in the objective function for parallel execution.
- Whether migration-based GA (using MigrationInterval and MigrationFraction) is a good alternative in my case.
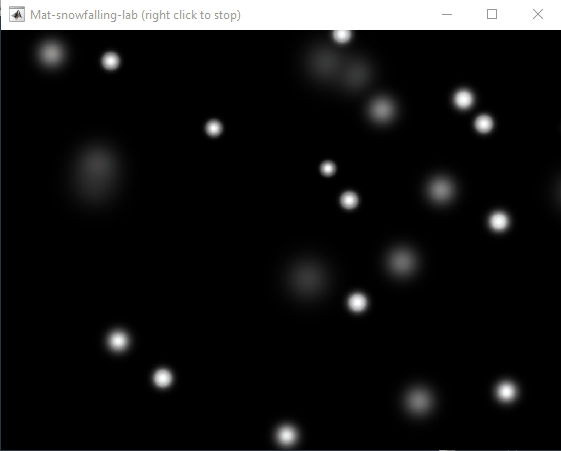